The maths of affiliate marketing
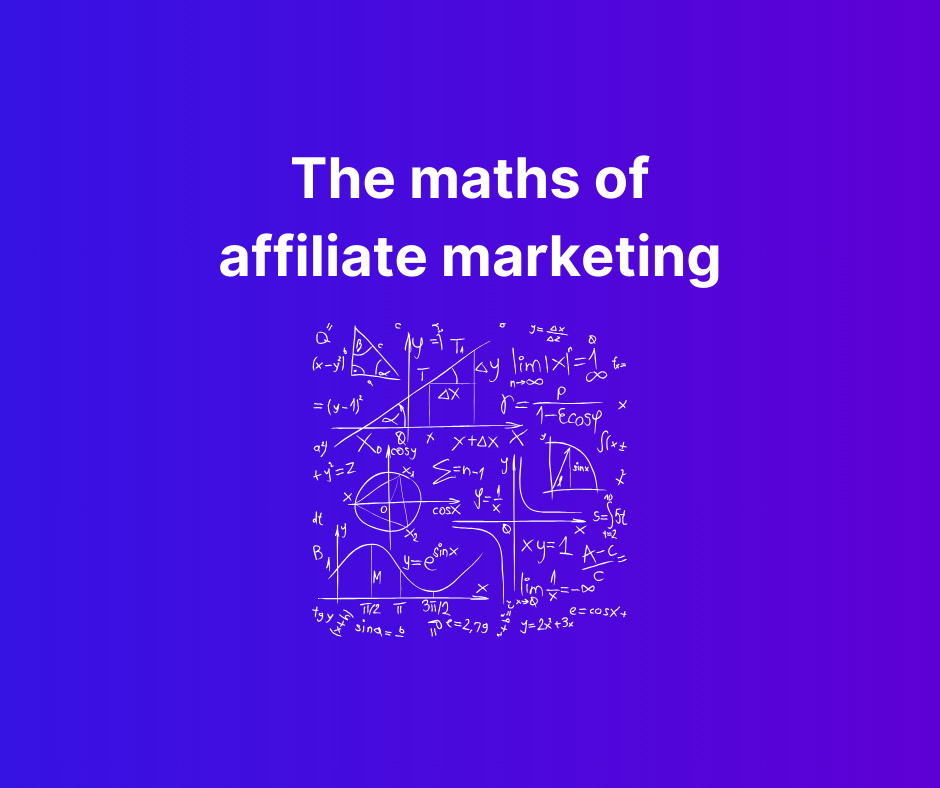
Maths in Marketing? Don't Panic!
Yes, numbers play a crucial role. So does statistical analysis.
The world of affiliate marketing is heavily reliant on data, particularly when it comes to paid advertising.
A typical affiliate marketing campaign involves multiple points where costs, conversions, and revenue intersect, all connected by mathematical relationships.
Whether it’s CPC, EPC, CVR, ROI or CPM, you need to understand what these terms mean and how they relate to each other, as it also helps you understand which levers you need to push and pull to drive things into the green.
Before we get to formulas, let’s outline some basic concepts and metrics you’ll want to think about as a budding media buyer.
Starting with the traffic source – advertising expenses
These can be calculated per view (CPM), per click (CPC), or sometimes per specific action (CPA).
Most often you’re going to be dealing with traffic sources that charge on a per impression or CPM basis.
You’re paying per impression, and their goal is to maximise how much money they make as a business – i.e. their revenue per mille (RPM).
If you're bidding on a per-impression basis, your banner's click-through rate (CTR) determines your effective cost per click (eCPC), i.e. how much you pay on average for each click that happens.
Fun fact: even on traffic sources where you bid in a CPC mode, chances are, behind the scenes, they are actually still focusing on their revenue per mille (RPM) and trying to maximise this.
This is how they offer multiple bidding modes.
Thus in a CPC mode, your click through rate matters more, as it directly impacts how much money the traffic source makes (and they care about this, a lot).
Moving to your mid-funnel content:
How well do your banners and landing pages perform in terms of click-through rates?
For each lander you are testing, what’s the effective revenue per view of that page? What about its ultimate conversion rate?
If you have multiple pages in a funnel, what is each of their clickthrough rate and the ultimate rate of users getting to the offer?
At the affiliate network level:
What's the offer conversion rate, payout, and earnings per click?
What's your average revenue per incoming click?
How does this fluctuate based on the original banner or landing page used before the person got to the network?
What's the effective earnings per click (EPC) for Landing Page A? Or Banner C?
How do these metrics stack up against each other, and what do they indicate about your profitability and return on investment (ROI)?
These are questions every marketer grapples with during each campaign.
It's essential to familiarise yourself with these terms and grasp how they impact your bottom line.
More importantly, understand how you can improve these metrics!
Now, let’s dive into the formulas, and later we’ll discuss some scenarios and using statistical calculators to understand potential and day-to-day optimisation decisions.
Formulas for our important metrics
Ok, this section is probably going to bore most of you.
You can skim past it, then come back to it later if you want to better understand what some formula or metric is.
At the traffic source level
CPM (Cost Per Mille)
- Definition: The cost to display an ad 1,000 times to users
- Formula: CPM = CPC × CTR × 10
- Example: If CPC = $0.75 and CTR = 2%, then CPM = $0.75 × 2 × 10 = $15
CPC (Cost Per Click)
- Definition: The cost to get a single user to click on an ad
- Formula: CPC = total cost / clicks
- Alternative Formula:: CPC = CPM / (CTR × 10)
- Example 1: If spend is $35.67 and total clicks is 87, $35.67 / 87 = $0.41
- Example 2: If CPM = $12 and CTR = 1.5%, then CPC = $12 / (1.5 × 10) = $0.80
CTR (Clickthrough Rate)
- Definition: The percentage of users who click on an ad, or clickthrough on a landing page
- Formula: CTR = (number of clicks / number of views) × 100
- Example: If 25 clicks and 8,000 views, then CTR = (25 / 8,000) × 100 = 0.3125%
Offer Maths
CVR (Conversion Rate)
- Definition: The percentage of users who take a desired action (e.g., downloading an app)
- Formula: CVR = (number of conversions / number of visitors) × 100
- Example: If 12 conversions and 200 visitors, then CVR = (12 / 200) × 100 = 6%
EPC/EPV (Earnings Per Click or View)
This can also replace “earnings” with “revenue”, and so E -> R
- Definition: The amount earned per action (click on an ad, or view to some destination)
- Formula: EPC = revenue / total clicks
- Alternative Formula: EPC = revenue × CVR / 100
- Example 1: If revenue is $415 and total visits is 2500, EPV is $415 / 2500 = $0.166
- Example 2: If Payout = $3.50 and CVR = 4%, then EPC = $3.50 × 4 / 100 = $0.14
CPA (Cost Per Action)
- Definition: The cost to get a user to perform a desired action
- Formula: CPA = actions / total cost
- Alternative Formula: CPA = CPC × 100 / CVR
- Example: You have spent $15 and got 7 total leads, so lead CPA = $15 / 7 = $2.14
- Example: If CPC = $0.35 and CVR = 3%, then CPA = $0.35 × 100 / 3 = $11.67
EPM (Earnings per Mille)
Note some platforms will state this as eCPM (a bit of an oddity given its not cost, but revenue)
- Definition: The amount earned per thousand views of an ad or page
- Formula: EPM = revenue / (views / 1000)
- Alternative Formula: eCPM = CTR × CVR × Payout / 10
- Example 1: If you’ve made $25 and have had 450 views to a page, then eCPM = $25 / ( 450 / 1000 ) = $55.6
- Example 2: If CTR = 2%, CVR = 5%, and Payout = $4, then eCPM = 2 × 5 × $4 / 10 = $4
Profitability Maths
Profit/Loss
Definition: The amount of money made or lost from an ad or campaign. There are many ways you can calculate this from direct metrics, other than just the obvious and basic “total revenue - total spend”. Look at these closely to notice how profit depends directly on the spread between key cost/revenue metrics.
Formulas:
- Profit = (EPC - CPC) × Total spend on ads / CPC
- Profit = (EPC - CPC) × Total click count
- Profit = (eCPM - CPM) × Total spend on ads / CPM
- Profit = (eCPM - CPM) × Total impression count / 1000
- Profit = (Payout - CPA) × Total spend on ads / CPA
- Profit = (Payout - CPA) × Total conversions count
- Profit = ROI × Spend / 100
Note how above for clicks you could use Total ad spend / CPC or Total click count – they give the same approximate value.
I prefer to use the total counts of clicks/impressions/conversions for this, because they are exact integers, whereas CPC, CPM etc. are derived/rounded values that originally came from the total data.
I also think it’s easier as it’s less items to deal with.
Examples:
- If EPC = $0.18, CPC = $0.15, and total clicks = 125, then Profit = ($0.18 - $0.15) x 125 = $3.75
- If eCPM = $22, CPM = $18, and total impressions = 4,000, then Profit = ($22 - $18) x (4000 / 1000) = $16
- If Payout = $5, CPA = $3.5, and conversion count = 17, then Profit = ($5 - $3.5) × $17 = $25.5
- If ROI = 250% and Spend = $300, then Profit = 250 × $300 / 100 = $750
ROI (Return On Investment)
- Definition: The percentage of initial spend expected as profit. An ROI of 0% is break even, an ROI of 100% is doubling your money
- Formulas:
- ROI = (EPC - CPC) × 100 / CPC
- ROI = (eCPM - CPM) × 100 / CPM
- ROI = (Payout - CPA) × 100 / CPA
Examples:
- If EPC = $0.25 and CPC = $0.20, then ROI = ($0.25 - $0.20) × 100 / $0.20 = 25%
- If eCPM = $30 and CPM = $25, then ROI = ($30 - $25) × 100 / $25 = 20%
- If Payout = $8 and CPA = $6, then ROI = ($8 - $6) × 100 / $6 ≈ 33.33%
Note: High ROI is valuable only when paired with sufficient spend to create a good profit.
ROAS (Return on Ad Spend)
- Definition: The revenue generated per dollar of ad spend. This is used in Ecom a lot and sources like Facebook. The formula is a little different to ROI and 100% ROAS is break even, whereas 200% ROAS is doubling your money. ROAS is typically expressed as a ratio (e.g. 3:1) or a percentage (e.g. 300%).
- Formula: ROAS = Revenue / Cost
- Example: If revenue is $270 and cost is $180, ROAS = 270 / 180 = 150% or 1.5
Pushing and pulling levers for profit
Ok, so there’s a lot of metrics/abbreviations and maths to throw around.
But what do I actually do when running a campaign? What do I focus on?
So, firstly you should read my earlier article on split-testing if you haven’t done so already – you need to be testing the biggest levers first.
Which thing you are testing is going to matter a lot more than what metric you choose to look at when making a decision.
In general, those are the offers, creatives, landers (if present) and overall angle.
In my opinion, your “north star” metric for a lot of these should be revenue per view (or click in the case of ads).
If you have a funnel that goes creative > lander > offer, and you are split-testing 5 of each, chances are if you lock in the highest RPV element(s) at each step, you’ll get close to optimal profit.
There’s a catch though – sometimes the element with the best RPV in each step, in isolation, doesn't necessarily give the best end-to-end path.
Example: creative 1 > lander 1 > offer 1
– looking at creatives, landers and offers on their own, #1 is the best in each. Great.
However, creative 2 > lander 3 > offer 2
might actually have the best RPV when considering this combination exclusively.
That could happen because the creative 2 ad best aligns with lander 3, which best aligns with offer 2 – giving the best overall flow.
This is why we consider “funnels” and use tracking platforms like FunnelFlux where we can break down this flow and see the metrics at each step, in combination. But such analysis is a bit complex and best kept for later, when you have real data to work with.
At the beginning, keep it simple and focus on the basics.
Using statistics for making decisions
This would not be a very good maths article (or affiliate marketing one) if I didn’t dive into how to use statistics for making decisions.
By decisions, I mean which variants are the best during split-testing, and “cut” decisions on whether to keep an ad, zone, lander, or offer.
Now, note in this article I am NOT going to get into rules for testing offers, cutting campaigns and so on.
These are less about the maths and more about experience and workflow. I will cover that in a later article, likely in a campaign-focused workshop rather than these beginner theory articles. Some things are easier to understand when you’re getting your hands dirty.
Coming back to stats…
There’s two statistical terms you’ll encounter here that are useful:
- Binomial distributions
- Bayesian inference
No need to understand how these work, just that we can use them in our decision making processes.
Binomial distributions
The word “binomial” basically means having two terms.
In the scope of split-testing, we are considering two outcomes in a probability event – convert or not convert. Clickthrough or no clickthrough. Action or no action.
A binomial distribution describes the outcomes of a situation where:
- There's a fixed number of attempts or trials
- Each trial has only two possible outcomes (often called "success" or "failure")
- The probability of success stays the same for each trial (which would be our conversion rate)
- Each trial is independent of the others
Here's a simple real-world example to help illustrate:
Imagine you're flipping a fair coin 100 times.
For each flip:
- There are only two possible outcomes: heads (success) or tails (failure)
- The probability of getting heads is always 50% (0.5)
- Each flip is independent of the others
The binomial distribution would tell you the probability of getting a certain number of heads (successes) out of those 100 flips.
Let’s show this visually – a binomial distribution would look like this, where the data tells us the chance of getting X number of heads when we do those 100 coin flips:
Here you can see the chance of getting 50 heads (our 50% known chance) is the highest.
But there’s a lower but significant chance of getting 40 or 60 and so on. The further we go from our known chance rate, the lower the probability.
In essence, we are showing the expected spread in chance in the data.
The more trials we have, the tighter this distribution will become.
Let’s see what this looks like for 100, 1000 and 5000 trials with a 5% chance:
And conversely, for the same number of trials, the higher our conversion rate, the more absolute spread in the data (but lower relative % spread):
See above how the data looks wider, and indeed the width does get bigger – but the % goes down.
In other words if we cut out the same 50% chunk from the middle of each peak, that chunk is getting smaller and smaller on a % basis.
What I want you to take away from this is two things:
- The more trials and conversions you have, the tighter the distribution in your data
- More data = more confidence in decisions
- The higher the conversion rate, the bigger the absolute spread in the chance, but smaller relative % change
- Low conversion rates increase the relative spread of the chance
So… what can we now do knowing a little bit about binomial distributions?
Using binomial distributions to make decisions
We can use binomial maths to take the performance of some item (a lander, creative, zone etc.) and ask the question “what is the likelihood that this meets my desired conversion rate”.
This lets us take a creative, lander, offer or something else and given a certain number of trials/successes, checking the chance that it will meet some goal conversion rate, within a certain confidence level.
We can use this for making “kill or keep” decisions.
For example, we can take a set of landers, with the following conversion rates (assuming a single offer payout here).
Based on the offer payout, I need the landers to make a 2.20% conversion rate to meet my profitability goals.
Here’s my current split-test data:
Based on the data above, which ones statistically have the higher chance of meeting that 2.2% goal?
They are all below the goal, so it’s not quite so easy is it?
Basic logic would lead people to think the higher conversion rate = higher chance, pick those.
But this is not a statistical approach. We instead can consider a binomial distribution for each lander, with a certain confidence, and look at the upper/lower limits of conversion rate to expect (if the experiment were to continue). From that, we can make better guided decisions.
Here’s the table again, but now with binomial confidence interval data added, with a confidence level of 90%.
Was this the results you expected when you first glanced at the data? Probably not.
Notice how the middle two both have conversion rates of ~2% so are closer to our goal.
However, they have more visit/conversion data, which makes the spread in that conversion range smaller. Because they are below our target, that worked against them.
On the other hand the first lander has the lowest conversion rate by far.
But it has the least data, so the minimum/maximum expected conversion rate is wider, and at this stage we can’t yet confidently cut it.
Now, when running real campaigns it can be a bit trickier, with the split-testing of multiple offers and such (making the needed conversion rate itself a bit unclear), but you can always simplify things down when focusing on a specific metric.
You could do the same with ad CTR, lander CTR, or offer conversion rate, where you have some “rate of something” i.e. a fraction and you want to calculate whether it will, over time, potentially be high enough to hit your target.
If it’s already above your target, of course the decision is to keep it, as even though long-term it might go below the target… you should never kill off something that’s profitable/working right now.
You would never stop something just because it might perform worse later.
Bayesian Inference
Binomial distributions are useful for a single experiment in isolation with a binary outcome.
Bayesian inference is much better when you want to compare multiple variants in an experiment and decide which one is most likely to win long-term.
This is more intuitive to most – you want to take several landers side by side and ask “which one of these is the best, statistically”.
In traditional (frequentist) A/B testing, you typically wait until you've collected a predetermined sample size before drawing conclusions.
Bayesian split-testing, on the other hand, lets you continuously update your probability estimates as new data comes in.
One key advantage of the Bayesian approach is that it allows you to make decisions at any point during the test, based on the current probability of each variant being superior.
This can lead to faster decision-making, especially when one variant shows clear superiority early on.
Now, if you have used any split-test calculators - like the one here or here - you are probably already familiar with what this data looks like. You have probably also seen bar indicators like this before:
Remember the distribution graphs I showed earlier? These bars are just a short way of representing those, and we are looking at the overlap in their distributions:
Here we can see that, if we look at the 95th percentile of each distribution (basically 95% of the area), and their overlap, the UPPER edge of the first (blue) distribution doesn’t reach the mean conversion rate of 2.12% of the second variant B.
Likewise the LOWER edge of the second (orange) distribution doesn’t touch the mean 2.00% conversion rate of the first variant A.
So we can now confidently say, with 95% confidence, that variant B is better than variant A.
Bayesian inference analysis is useful for doing the above but with many variants, giving you data like this:
Using the tool above, if you scroll further down you can see the graphing of distributions:
Above it says no winner can be declared yet. Looking at the graph above, this is visually quite intuitive right?
You can tell at a glance that the lowest conversion rate variant still overlaps quite a bit with the one having the highest mean conversion rate.
So… where to go from here.
The point of this all – you can use Bayesian inference analysis to make decisions on whether to cut or keep certain variants, whether it’s creatives, landers or offers.
And you SHOULD. Especially for things you pick and control, like landers and offers.
For items like zone/placements IDs on traffic sources that have them, it would however be cumbersome to run such analysis on all of them – and generally there you are not looking to pick a winner.
You want to cut what doesn’t work and keep what does, which could be thousands of items or more.
You would keep placements with 10% ROI even if there are ones with 1000% ROI – it’s not a winner/loser game where you want to keep only the best.
Not so for landers where you definitely want to keep the best and cut the low performers.
So, TL;DR:
- Use binomial distribution calculations for cut/kill decisions where you have a goal rate of something (CTR, CVR etc.) and you want to make guided decision on whether you have enough data to cut or kill them
- Use Bayesian inference testing when you want to compare multiple variants of something you control and decide which ones to keep based on likelihood of them being the best long-term performer
Note in both cases you are dealing with rates, not revenue per view or profit. So you need to take into account what might be happening behind the scenes, e.g. behind landers you may be split-testing offers.
You can simplify your testing by assuming all landers are getting the same blend of conversions from all offers (always drill into your data to confirm this).
Hopefully from this you now have a stronger understanding of the maths of affiliate marketing!